

The CLT tells us that about 68% of sample means should occur within one standard error above or below the distribution mean, and 95% should occur within two standard errors above or below. If you have 100 iterations, the standard error is 20/√100 = 2. For example, with RiskNormal(655,20) the standard deviation is 20. One SEM is the standard deviation of the input distribution, divided by the square root of the number of iterations per simulation. The Central Limit Theorem of statistics (CLT) answers this question with the concept of the standard error of the mean (SEM). The question naturally arises, how much separation between the sample mean and the distribution mean do we expect? Or, to look at it another way, how likely are we to get a sample mean that's a given distance away from the distribution mean? With enough iterations, Monte Carlo sampling recreates the input distributions through sampling. A problem of clustering, however, arises when a small number of iterations are performed.Įach simulation in or RISKOptimizer represents a random sample from each input distribution.
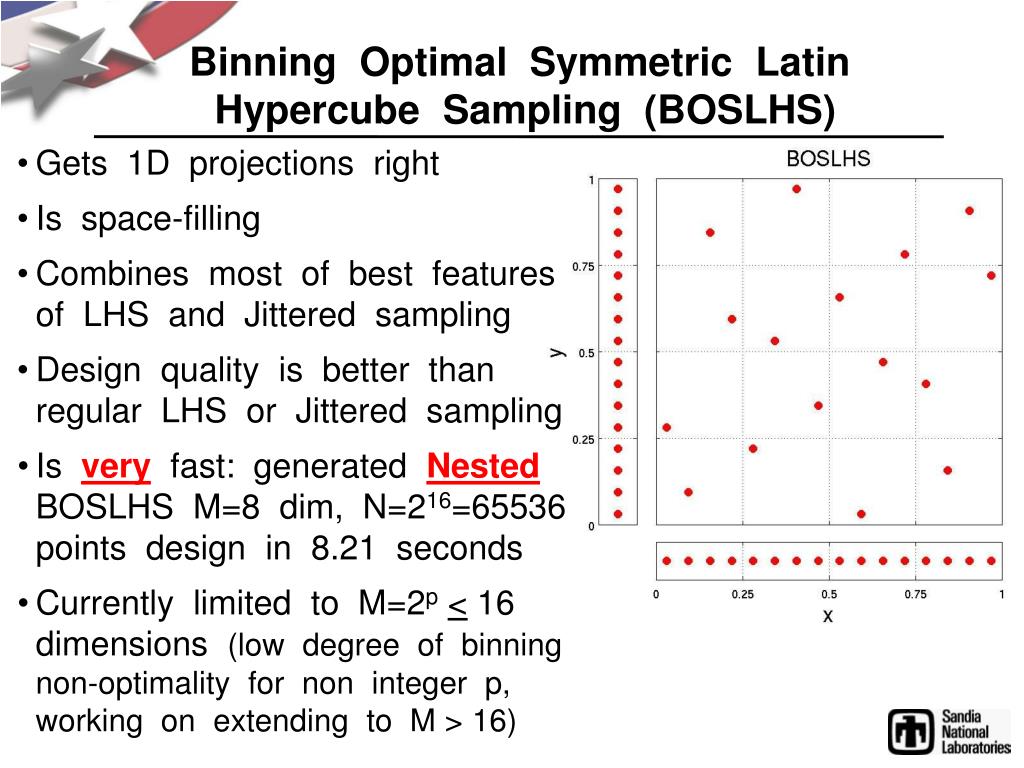
Monte Carlo sampling refers to the traditional technique for using random or pseudo-random numbers to sample from a probability distribution. Monte Carlo sampling techniques are entirely random in principle - that is, any given sample value may fall anywhere within the range of the input distribution.
